Limitations and benefits of machine learning
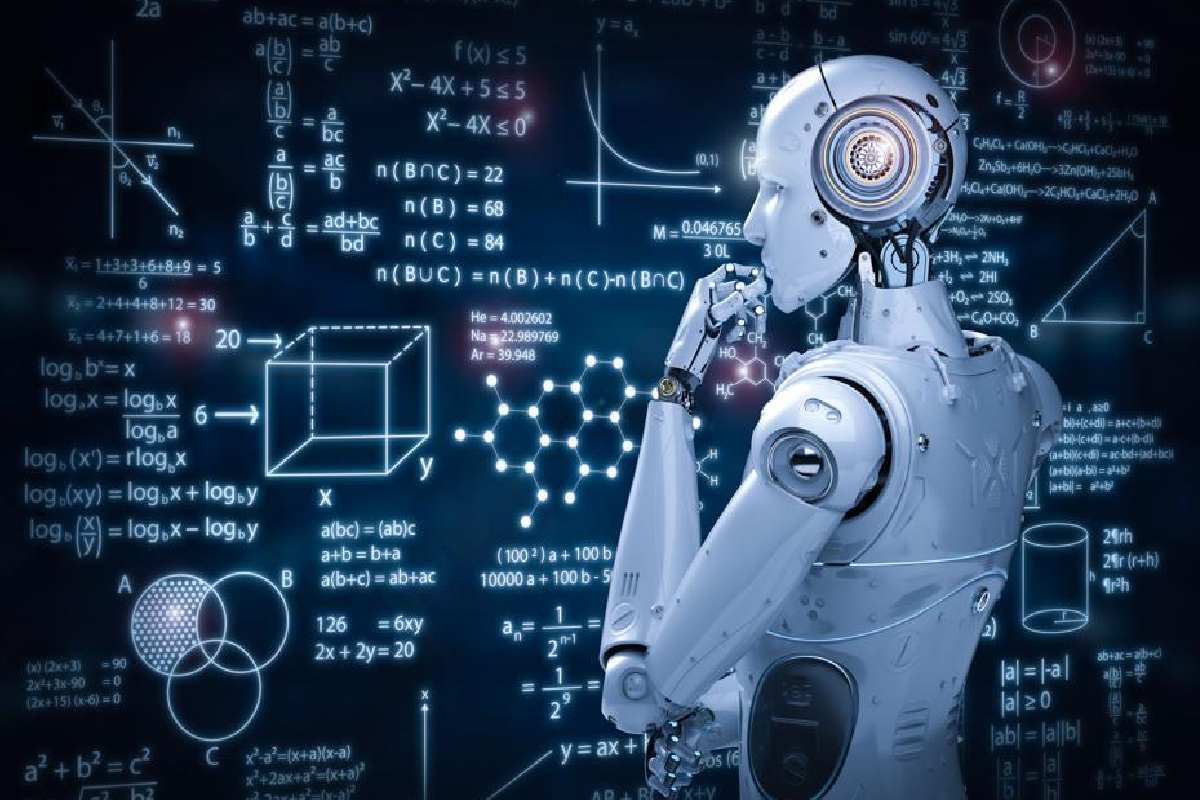
We live in a time when the computer takes up more and more space in our lives. And if at the beginning of the century it was a luxury and was used mainly for work and computing, today even ordinary phones are mini-computers, much more powerful than those that were in offices at the beginning of the 2000s. Why are there telephones! Even some refrigerators are able to determine what products are lacking in them, and independently place orders via the Internet.
Computers are in charge of our finances and security and solve everyday problems. Moreover, today you will not surprise anyone by the fact that you can talk to a computer, and sometimes a virtual interlocutor is more interesting, witty and understandable than a living person. And this happens because he, this interlocutor, has the ability to self-study, that is, each of the hundreds of thousands of dialogues that he conducts in the same period of time is at the same time a lesson for him. This is how artificial intelligence learns new words, tasks, reactions, jokes, sayings, and verbal expressions. And now sometimes it is not clear with whom you are communicating: with a living person or with a computer.
For ease of perception, types of machine learning ( machine learning agency) are usually divided into three categories:
- supervised learning;
- unsupervised learning;
- reinforcement learning
Table of Contents
Supervised Learning:
This type of learning is identical to the process of learning “everything in the world” by a child; the algorithm itself acts as a child. The data that give the algorithm for analysis already have the correct answer. Based on this, it should be said that the purpose of this action is to teach the algorithm not only to find the correct answer but also to understand what and why. The result is the ability to build correct forecasts and models.
Unsupside learning:
Is a machine learning method in which you don’t have to control the model. Instead, you need to let the model work on its own to discover the information. This is mainly true for unlabeled data.
Reinforcement learning:
The principles of reinforcement learning are borrowed from psychological experiments: the machine needs to take one or another action in different scenarios. Each action entails certain consequences, both short-term and long-term. The algorithm must analyze all possible options for further events and choose the optimal action at the present time.
Machine learning tools are used in the following stages:
- collection and preparation of data;
- building a model;
- training and deployment of applications.
Limitations and Cons of Machine Learning:
The first disadvantage is that the car lacks any kind of ethics. When we teach the machine something unusual and antiethical, the consequences can be completely unpredictable.
Unfortunately, a huge number of machine learning algorithms work not for the good of society, but for the good of their creators, observing only their personal interests.
False correlation is when things that do not depend on each other behave as much as possible in the same way and, as a result, one gets the impression that they are somehow connected.
Only feedback loops are worse than false correlations. This is when the decisions of the algorithm affect reality, which, in turn, further convinces the algorithm of its point of view.
Poisoning is an impact on the learning process. But you can also deceive a ready-made, properly working mathematical model, if you know how it works. For example, a group of researchers managed to learn how to deceive the face recognition algorithm using special glasses that make minimal changes to the picture and thereby radically change the result.
Review Limitations and benefits of machine learning .