Application of Data Science in the Finance Industry
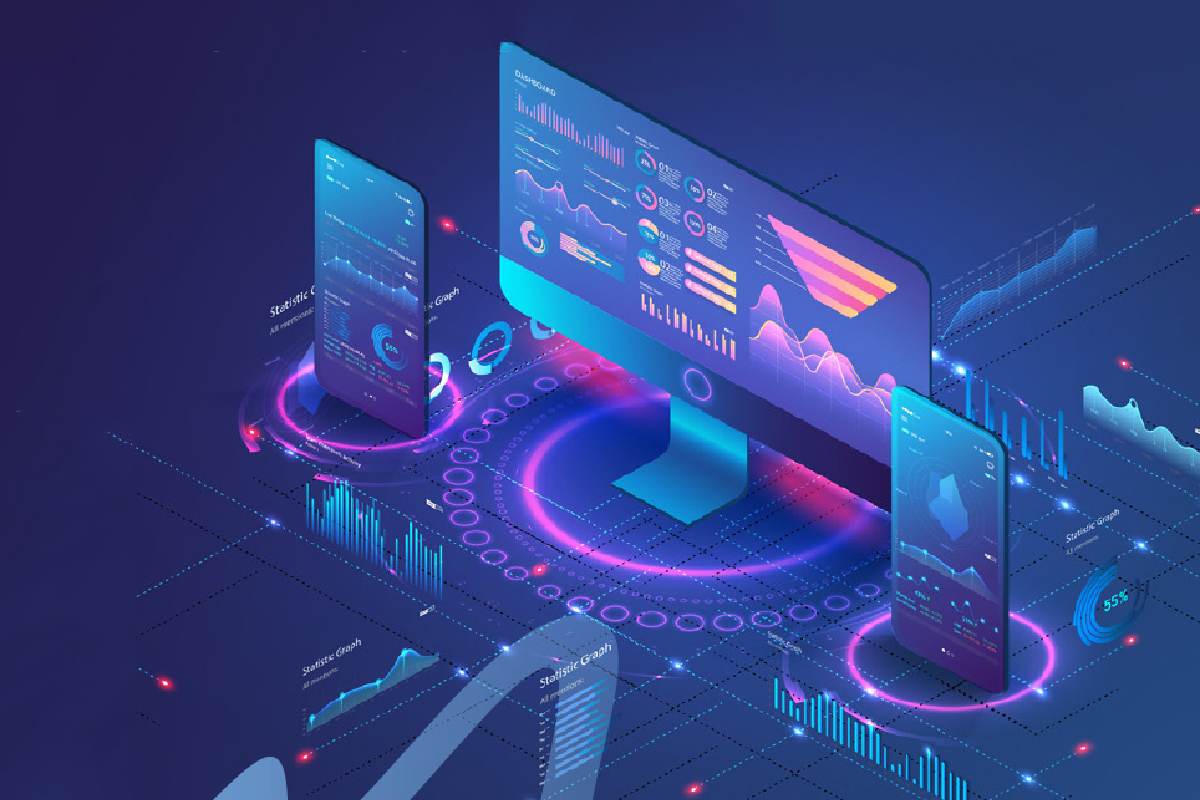
Finance is the motivating factor behind so many industries since it dictates everything from a company’s inception to its growth. As a result, financial management plays an important role in every sector, especially financing and banking. Financial management is intrinsically related to data, and without information, certain financial operations may be nearly impossible to accomplish. Data science applications in the financial sector are crucial since finance and data are integral. Let’s take a closer look at data science in finance.
Data science encompasses a variety of disciplines in which statistical and scientific methods to data are used to get insights. This data is critical for strategic decision-making. Because of the volume of data available today and greater accessibility to powerful processing capability, data science in finance has effectively been embraced to open further possibilities for data-driven decision-making.
If you wish to expand or start your career in this field, you can opt for a Great Learning data science online training program.
Table of Contents
Risk Analytics
Every organization has risk that must be assessed to be controlled on time. The financial sector’s risk assessment and management methodology includes procedures ranging from risk identification to risk mitigation. Financial crises, payment defaulters, or any other activity in the ecosystem might all pose risks. To save money, preserve their reputation, or limit their legal exposure, businesses must identify and manage risks.
Credit card transactions, credit reports, price variations, trade data, and other financial data are all recorded. Data science course is used to analyze these data sets to identify points of concern and take the required precautions to reduce the risks. Data science enables risk managers to analyze large data sets and gain adequate insights.
Financial Fraud Detection
Tax avoidance, insurance claims, and identity fraud are all examples of financial fraud. For organizations, tracking fraudulent potential and taking precautions to prevent loss is a top issue. The implementation of data science in finance has improved, resulting in more efficient algorithms that can identify the likelihood of fraud much earlier.
Fraud prevention becomes considerably more efficient when huge data is analyzed using data science, allowing businesses to intervene in real-time. Unusual variations in credit card activity or banking transactions may be flagged so that necessary action can be taken. This aids the firm in safeguarding a customer’s information and the organization’s reputation and operations.
Data science may also be used to prevent fraud in insurance claims. To understand patterns, data from previous claims are examined and analyzed, and this will be used to identify potential bogus claims.
By lowering false positives, boosting the identification of true fraud, and providing resources to manage it, data science has enhanced the effectiveness of fraud detection systems in financial institutions.
Consumer Analytics
The requirement to adapt to changing customer behavior is at the heart of the desire for predictive consumer analysis. Consumers today are much more informed and empowered, with higher expectations. An organization must know these clients’ tastes and lifestyles to respond favourably to them. An organization’s ability to forecast future behavior of the customers and provide relevant offers to them is a greater understanding to the customers.
Predictive consumer analysis is enabled by data science techniques, which aid in raising response rates, returning consumers, and profitability. These assessments also assist in improving the efficacy of their promotion initiatives. Clients who are most likely to respond positively to offers receive ads customized using such knowledge. With fewer and more targeted targets, advertising expenditures are also lowered.
Consumer insights may also keep customers from migrating to a rival. This information may be used to forecast their future activities and reply with appropriate offers. They can also assist in determining client lifetime values.
Customer Data Management
Consumer data management is crucial for a company’s success in today’s competitive business climate. Using big data analysis and data science, businesses may learn nearly anything about a customer important to their business.
Consumer buying behaviour and ethnicities are increasingly available to businesses. This information may be used to deduce marketing trends and predict customer behavior. Customer data management provides a comprehensive perspective of customer behaviour and aids in data-driven decision-making.
Client data management implemented correctly may significantly enhance customer retention and satisfaction, resulting in increased profitability. The analysis of current consumer data benefits financial organizations the most, and customers having a greater future value may be treated individually to maximize financial gains.
Real-Time Analytics
Timing is crucial in the financial industry, especially. As a result, real-time analytics are as critical as, if not more significant than, previous data analysis. Organizations can benefit from data science tools, and this aids business in better understanding their existing clients and improving their company image and connection with brand assets. They receive the possibility to enhance customer interactions and raise the likelihood of consumer loyalty.
Most financial organizations have legacy systems since they have been around for decades. The ability to fully leverage the potential of data is now possible thanks to the digital revolution and financial data science applications. Real-time analytics may assist offer a better product or service to consumers based on their preferences.
Algorithmic Trading
Algorithmic stock trading is the automated process of purchasing and selling stocks using complicated mathematical formulae. In stock trading, data science prediction models are applied, and these models utilize previous data to forecast future stock market happenings.
To optimize the profits of their stock investments, market participants employ a variety of trading tactics. To determine the profit margin of the equities, they analyze economic statistics, corporate data, and the most recent news about the firm. Fund managers attempt to forecast stock values and trade accordingly.
Algorithmic data science-based trading takes advantage of computing power and data accessibility. Certain factors are considered, such as time, volume, and share price.
Conclusion
Finance professionals use data science in several areas more than those described above. Data science and machine learning have been advancing in recent years, assisting businesses in automating their administrative operations and improving the value proposition they can provide to their consumers while also improving their bottom line.
When tackling corporate challenges, data-driven decision-making has yielded the best outcomes. You, too, can learn to make data-driven decisions by taking Great Learning’s data science online classes.